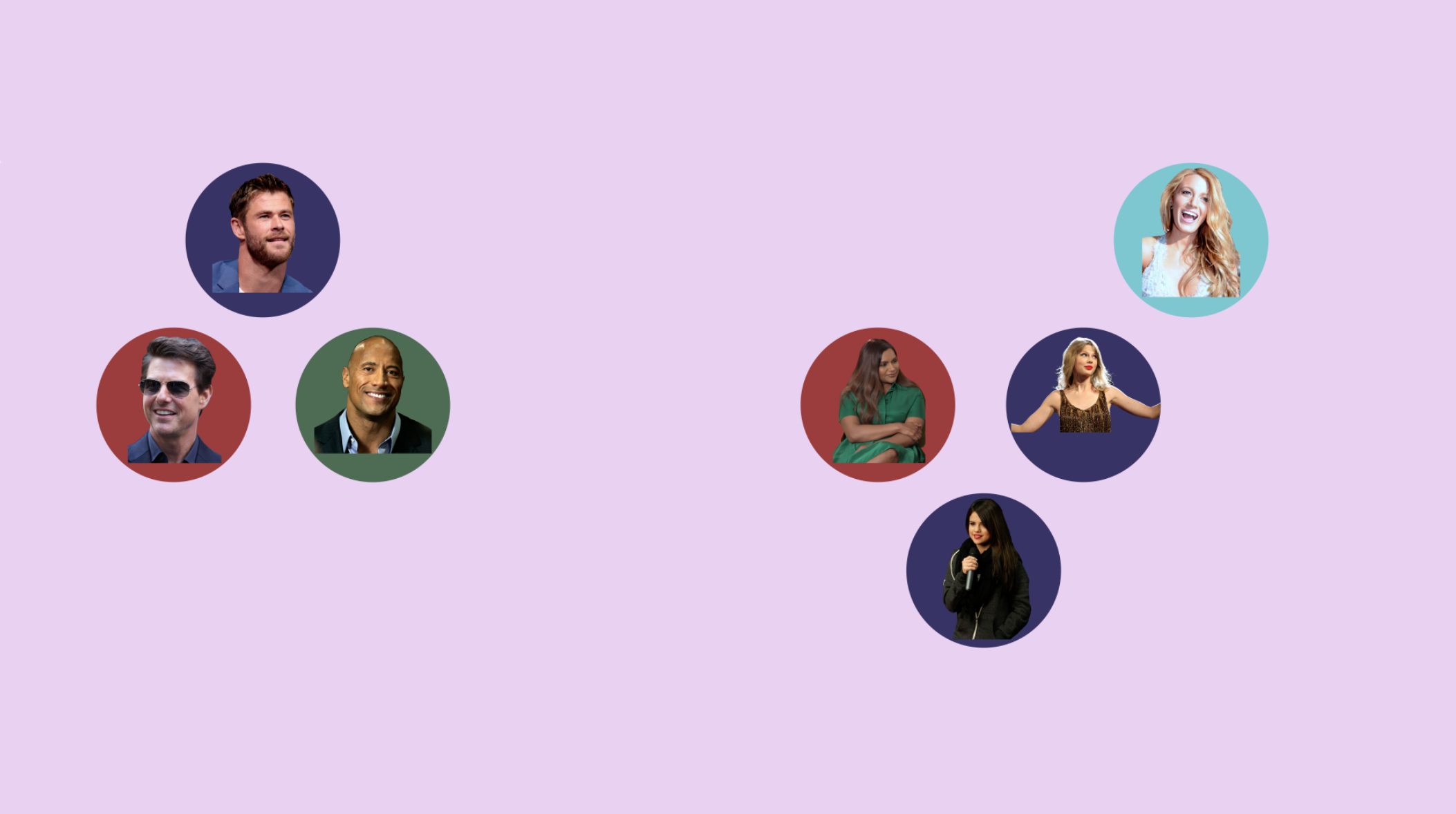
By Jillian Mock
In 2018, it’s basically impossible for me to imagine applying for a job, finding a date or bragging about my latest vacation without some assistance from the internet. Love it or hate it, many social interactions are online, and the digital and physical worlds create a strange, combined reality.
In some ways, this is good. The internet can be a level playing field, where anyone’s voice can be heard and elevated by millions of other people. There’s a darkside to that—trolls and hate groups can have their voices amplified, too. Social media is full of nonverbal shouting.
But some of the problems with our digital way of life are largely invisible to us. Behind the scenes, algorithms determine what we see and when we see it on a given Internet platform. At first glance, it might seem these unemotional machines are the best, most unbiased arbiters of our online social interactions. But these algorithms pick up on our biases and amplifies them. The result is a digital world that picks winners and losers from behind the scenes—and those winners tend to be white and male.
The size of your network and your visibility on social media matters for all kinds of professional development opportunities. It can help you land a job, a book deal, a speaking gig, advertising dollars based on your social media presence and so much more. While numbers of followers on Instagram or Twitter may seem like a silly metric at first glance, limiting the number of followers you are exposed to can limit real world success. And what you see online also shapes what opportunities you know about out in the real world.
Let’s think about applying for a job. A 2015 study found Google was less likely to show a woman ads related to high-paying jobs than a man. In the study, the researchers had half the participants select “female” on their Google Ad Settings and the other half selected “male.” They then simulated looking for a job by visiting websites associated with job hunting to clue the algorithm in to what they were looking for. The male participants started seeing ads for career coaching to win a high-paying job significantly more often than the female participants.
Similar things can happen along racial lines. A 2013 study by Harvard researcher Latanya Sweeney found Googling historically African American names resulted in ads suggesting that person has been arrested more often than if you Googled historically European American names, even if no one by that name has ever been arrested before.
These are just two ways algorithms become biased, often to the benefit of white men. But how does this actually work? To take a look under the hood, let’s turn to Instagram.
In a recent study out of Columbia University, researchers found a “glass ceiling effect” when it came to gender and connections and influence on Instagram.
“People who are very popular with high engagement have in a sense more power to influence their peers,” says Ana-Andreea Stoica, a graduate student at Columbia and one of the researchers on the project.
To look at this platform, Stoica and her team pulled the demographic information from 554,000 different users on Instagram in 2014 and 2015. This was before Instagram introduced its “Suggested Accounts” algorithm, so the Columbia team believes this data represents organic growth, with people finding each other and linking and commenting on their photos without interference from a recommendation algorithm.
After pulling this data and determining the gender of each user, Stoica and the other researchers analyzed how many likes and comments each user received and who was liking and commenting on what. The researchers decided to focus on likes and comments as indications of engagement rather than followers, as likes and comments are much more active than merely accruing followers.
Without any algorithms in the mix, people gravitated naturally to groups of people like them, the study found. Women engaged more with other women through likes and comments and men engaged more with other men. But the group affinity was stronger in men, who were more likely to connect—again through likes and comments—primarily with other men. Women, while they connected more with other women, would also throw likes and comments to men.
This tendency, combined with the fact that men on average had more likes and comments on their posts, made it easier for men to rise to the top of the pack. Even without adding recommendation algorithms into the mix, it was more difficult for women to rise to be among the most liked and commented profiles within the sample.
“Initially men have more connections,” says Stoica. “And then they connect more with other men and over time… more men connect with other men, leaving the women to the side.”
Then the researchers implemented recommendation algorithms similar to the ones Instagram likely started using in recent years (the exact algorithms are proprietary information). They expected the algorithm would recommend women as connections more often because there were more female users to recommend in their sample (53 percent women and 47 percent men).
Instead, they found the algorithms picked up on existing patterns in the data. The algorithms assumed men were the most desirable connections because the minority population of men already had more connections and showed such a strong tendency for connecting with other men. As a result, these algorithms recommended men as new connections slightly more often than they recommended women, giving the men yet more opportunities to get higher levels of engagement on their posts than women.
“It’s a little bit weird because women are the majority, the users are 53 percent women,” says Stoica. “Once the algorithm was put in place, then this hierarchy shows even higher percentage of males at the top, much higher than you would expect and much higher than it was before.”
This trend was really clear in the most-influential, top 0.1 percent of users with the most likes and comments on their posts. Although the group of influential men was slightly smaller than the group of influential women (the high influence user pool was 48 percent men and 52 percent women), the men were recommended 74 percent of the time and women only 26 percent of the time.
“In a sense, the data exhibited a ‘glass ceiling effect’ that was amplified by these recommendation algorithms, not created by them,” says Stoica.
While there are lots of women on Instagram, it’s harder for women to rise to the very top of the most popular, most successful accounts. Stoica likens this to women in business—there are lots of women hired at lower levels, but fewer women hold management positions. In 2018, women hold only 14.6 percent of executive positions in U.S. businesses and only 4.6 percent of Fortune 500 companies have a woman as CEO, according to the Center for American Progress.
The real problem here, says Stoica, is that algorithms don’t just mirror discriminatory behavior. They amplify it. The algorithms pick up on the way people naturally cluster together and then make it easier for certain groups to attain more connections and higher levels of engagement on their posts. More engagement and connections translates into a larger reach to promote your products, advertisements, professional agenda and much more.
“If there was no community at all, if everyone connected to everyone in exactly the same way, then these algorithms would not be able to pick up on anything, they would not amplify any inequality,” Stoica says.
The solution, says Stoica, is to build better and more socially aware algorithms. But people are still trying to figure out how exactly to do that. “In that case, we’re not going to fix the inequality, we’re just not going to amplify it,” says Stoica. It’s not ideal, but perhaps it’s not the job of algorithms to help us overcome broader social inequality, she says. Maybe the best we can hope for with algorithms is to not make social inequality any worse.
For now, perhaps it’s enough for us to unmask the man behind the curtain, to know the internet operates a little differently for everyone. That way, as we apply for jobs and sell homemade hair care products and agitate for a more free and equal society in the real world, we can push for that in the digital realm, too.
Image Credits: Wikimedia Commons Dwayne Johnson, Wikimedia Commons Selena Gomez, Wikimedia Commons Blake Lively, Wikimedia Commons Tom Cruise, Wikimedia Commons Chris Hemsworth, Wikimedia Commons Tessa Thompson, Wikimedia Commons Anthony Mackie, Wikimedia Commons Paul Rudd, Wikimedia Commons Mindy Kaling, Wikimedia Commons Mark Ruffalo, Wikimedia Commons Taylor Swift, Wikimedia Commons Jennifer Lopez, Wikimedia Commons Rashida Jones, Wikimedia Commons Ryan Gosling, Wikimedia Commons Heart Image, Wikimedia Commons Curved Arrow, Jillian Mock Instagram Screenshot.